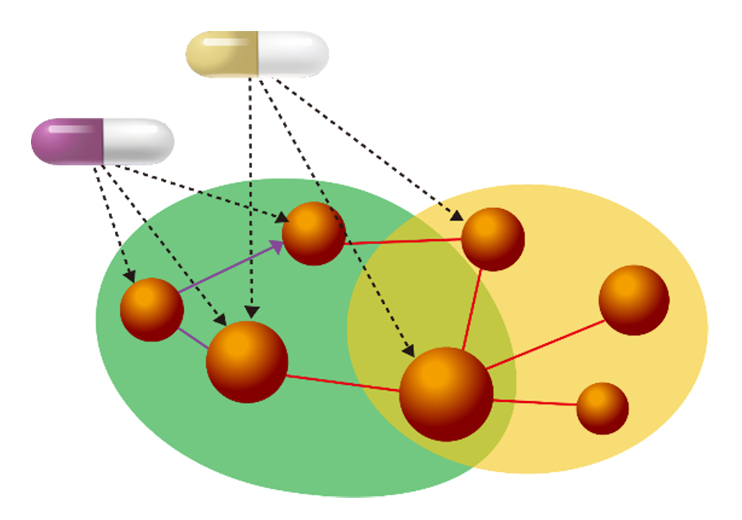
The integration of artificial intelligence (AI) technology with the profound knowledge of traditional Chinese medicine (TCM) presents an ambitious goal: harnessing the unique strengths of TCM to devise novel strategies for preventing and treating major diseases, such as cancer. TCM has a long-established tradition of employing non-invasive diagnostic techniques that can detect subtle signs of disease formation. These techniques include facial, tongue, and pulse diagnosis.
By fusing AI with TCM, our researchers aim to revolutionize the way these diagnostic methods are applied. AI's computational power and analytical capabilities allow for the systematic and precise analysis of vast amounts of patient data, leading to more accurate and reliable diagnoses. AI algorithms can discern patterns and correlations that might be difficult for human practitioners to identify, thereby minimizing errors and biases that could be introduced through human interpretation. Through the use of AI, TCM's diagnostic approaches can be standardized and generalized, making them more widely accessible and applicable across different patient populations. This standardization enhances the reproducibility and reliability of TCM's "preventive treatment system", enabling healthcare professionals to implement consistent and effective preventive measures for various diseases.
Related articles:
· Zhang P, Wang B, Li S*. Network-based cancer precision prevention with artificial intelligence and multi-omics. Science Bulletin, 2023;S2095-9273(23)00342-0
https://doi.org/10.1016/j.scib.2023.05.023
· Zhang S, Yang K, Liu Z, Lai X, Yang Z, Zeng J, Li S*, DrugAI: a multi-view deep learning model for predicting drug–target activating/inhibiting mechanisms, Briefings in Bioinformatics, 2023; 24(1), bbac526
https://doi.org/10.1093/bib/bbac526
· Zhang P, Li S*. Human cross-tissue cell atlases: unprecedented resources towards systematic understanding of physiology and diseases. Signal transduction and targeted therapy. 2022; 7(1): 352.
https://doi.org/10.1038/s41392-022-01201-w
· Ding Q, Hou S, Zu S, Zhang Y, Li S*. VISAR: an interactive tool for dissecting chemical features learned by deep neural network QSAR models. Bioinformatics, 2020; 36(11), Pages 3610–3612.
https://doi.org/10.1093/bioinformatics/btaa187
· Guo Y, Nie Q, MacLean A, Li Y, Lei J*, Li S*. Multiscale modeling of inflammation-induced tumorigenesis reveals competing oncogenic and oncoprotective roles for inflammation. Cancer Research 2017;77(22):6429-6441
https://doi.org/10.1158/0008-5472.CAN-17-1662